Our lab focuses on improving and developing methods in privacy-preserving machine learning, which aims at facilitating data analyses without sacrificing privacy. Our research program has three main objectives: to improve the trade-offs between privacy and accuracy; to advance differentially-private synthetic data generation techniques; and to study the interplay between differential privacy and other emerging characteristics such as interpretability, fairness and causality. Our work is particularly relevant to applications in healthcare, where analyzing privacy-sensitive data is a must for patients and clinicians alike. The technological solutions that we are working toward will help reduce the sensitivity of algorithms to sparse data points, facilitate the sharing of data between healthcare service organizations and improve systems used for human decision-making support through a focus on fairness, causality and interpretability.
Our group photo (July, 2023).
Left to Right: Kamil, Mi Jung, Michael, Saiyue, Justin, Margarita, Charlie, Frederik.
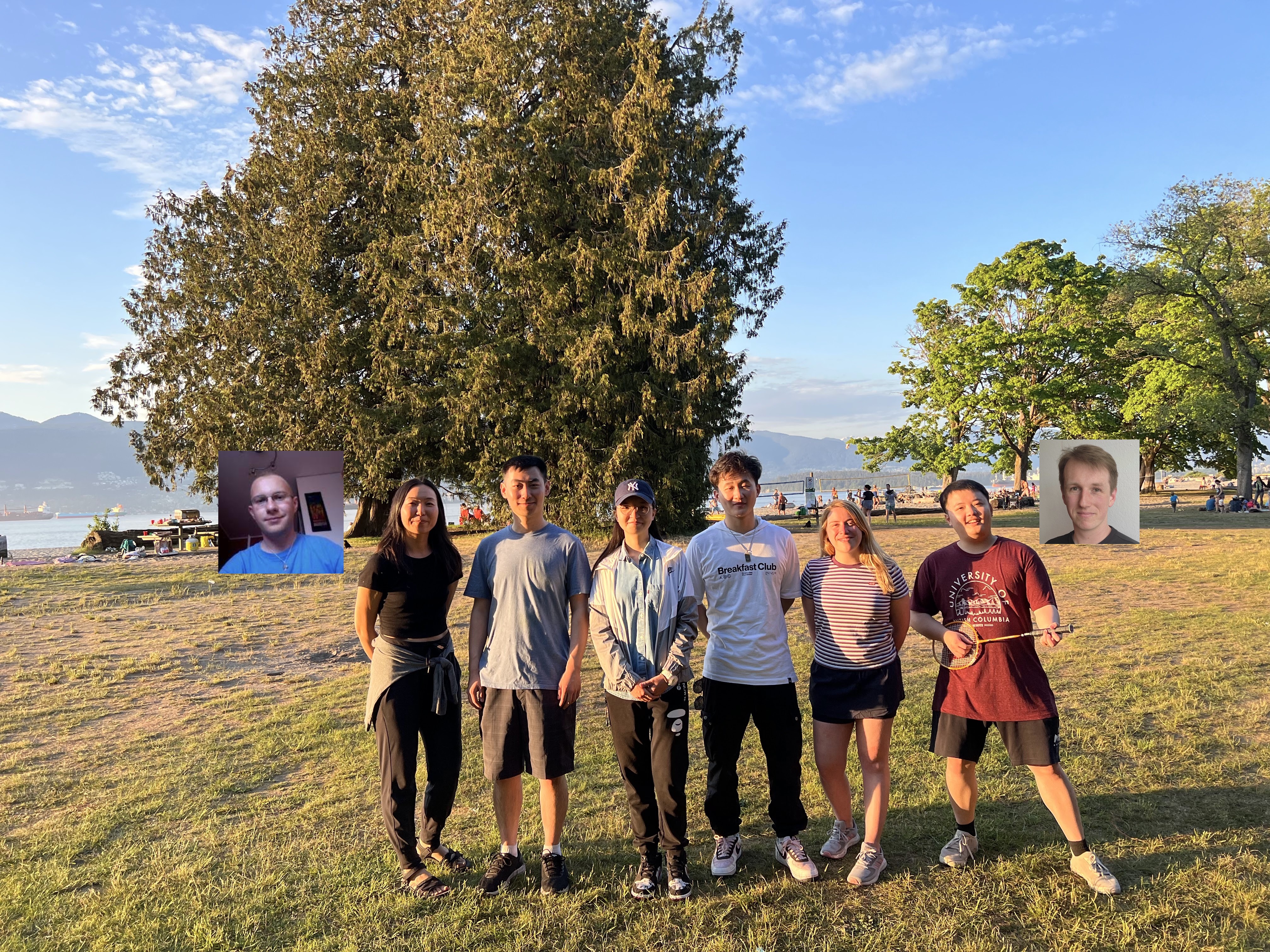